SC1: Emerging methods for data processing and acquisition
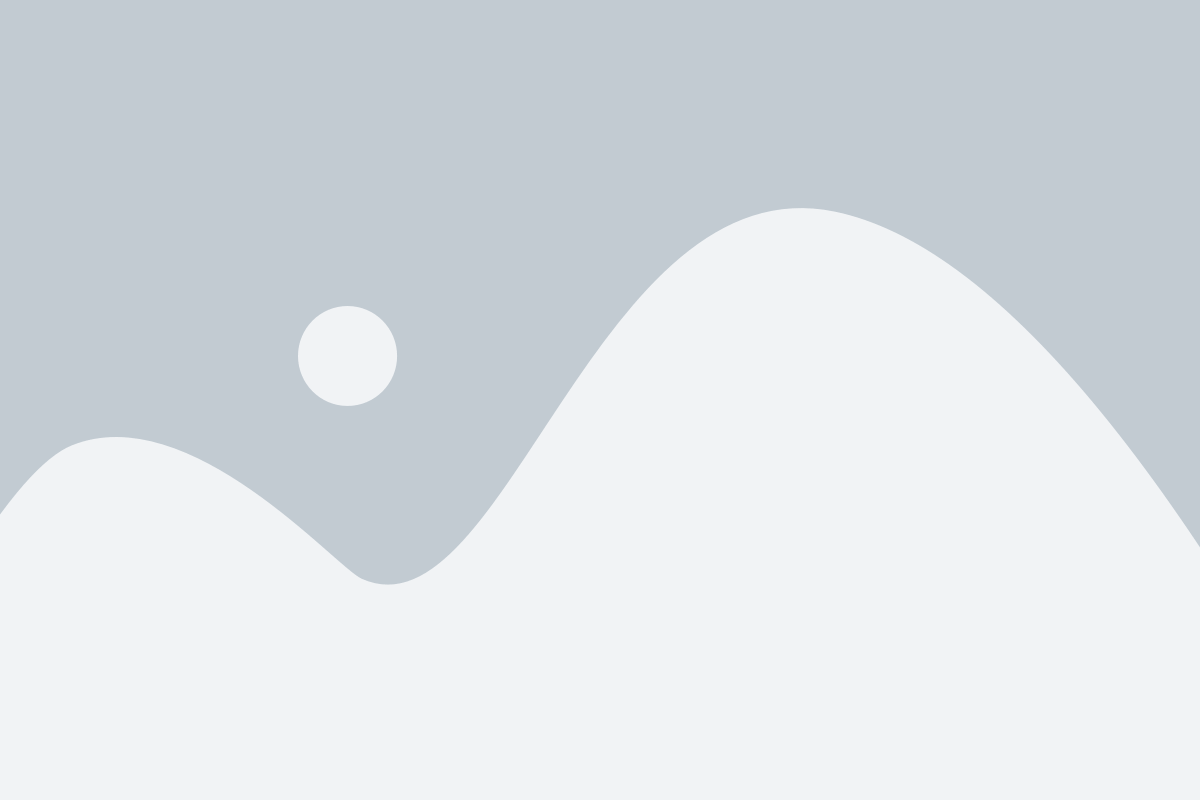
Maxime Moreaud
IFPEN, Solaize et MINES ParisTech, Fontainebleau
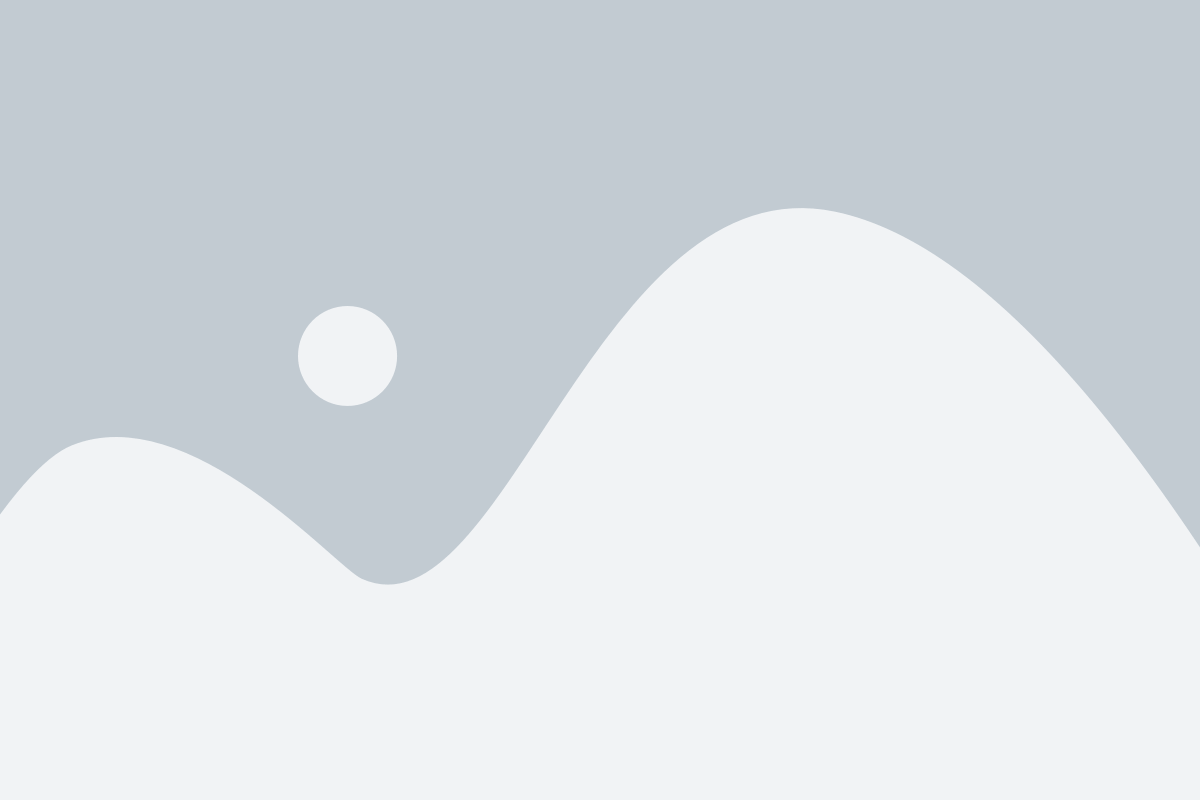
Christophe Zimmer
Unité Imagerie et Modélisation, Institut Pasteur et UMR 3691, Paris
Deep learning has changed the game of imaging data processing and strongly impacts the field of microscopy. In imaging, it is generally a deep convolutional neural network that searches for similarities in images with different degrees of accuracy for different scales of observation. This similarity search then allows to solve a large number of problems. For microscopy, these new approaches revolutionize the detection of objects of interest, classification, segmentation, noise reduction, resolution enhancement or modality fusion. They allow both to increase the quality of images or to extract more quantitative information. Learning methods feed on data, which can be obtained experimentally or constructed numerically. Despite their power, these methods also have limitations that raise important issues, such as the generation of artifacts when deviating from the training data or the difficulty in interpreting the decisions of neural networks. In this symposium, we will focus on these new approaches, their implications for microscopy methods and applications, as well as more classical approaches to extract more information from images. We are also interested in emerging methodologies related to acquisition to improve resolution and/or speed of image capture.
Keywords: emerging methodologies, data mining, deep learning, image processing.
Invited speaker
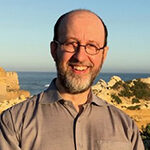
Hugues Talbot
CVN-INRIA, Centrale Supelec, Saclay
Une revue des méthodes de segmentation par apprentissage automatique